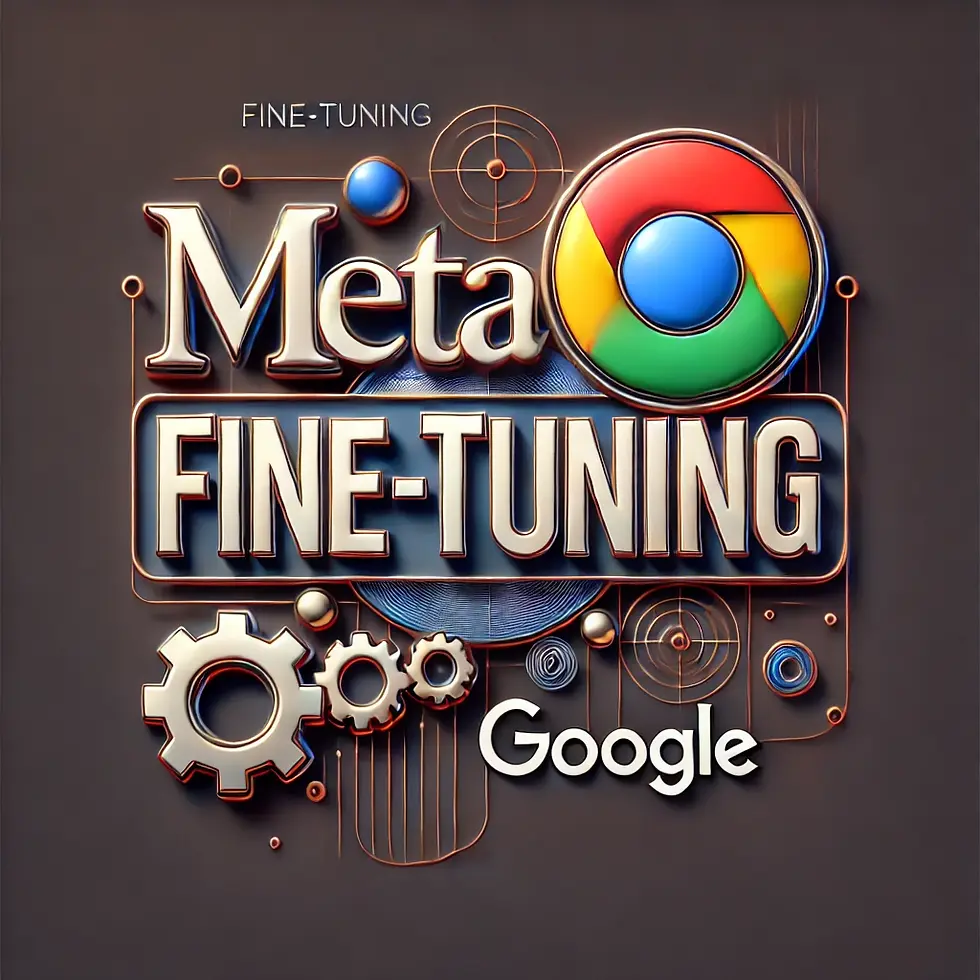
As AI technology continues to evolve, many CEOs are questioning whether the practice of fine-tuning models is still relevant. With small models today performing at the same level as large models from just a few years ago, fine-tuning, which can be time-consuming and complex, might seem less essential. However, there are specific cases where fine-tuning remains not only necessary but critical for achieving optimal outcomes.
When is Fine-Tuning Necessary?
While out-of-the-box models, especially those powered by large language models (LLMs), are highly effective, fine-tuning remains vital when:
Customization for Specific Tasks: If your application requires industry-specific language or complex task execution, fine-tuning can help tailor a model to meet your exact needs. For instance, financial institutions or healthcare providers often need models that understand their particular jargon and processes.
Enhancing Performance with Real-World Data: Fine-tuning allows models to learn from actual client interactions. By incorporating live user data, your AI can adapt to the unique behaviours, preferences, and needs of your customer base. This is particularly important in industries like customer service or product recommendation, where personalized user experiences drive success.
Avoiding Risky Decisions: Fine-tuning helps ensure that models not only perform tasks but also avoid undesirable behaviours, such as generating biased responses or incorrect recommendations. Regular fine-tuning using real-life data helps the model "learn" how to make fewer mistakes.
When to Skip Fine-Tuning
However, fine-tuning isn’t always necessary. If you’re using a model for more general tasks, or if the model’s out-of-the-box performance is sufficient for your application, the time and resources needed to fine-tune may not offer a substantial return on investment. For many use cases, retraining or fine-tuning can be delayed until you have enough data to justify the effort.
The Fine-Tuning Process
Fine-tuning isn't just about tweaking a model; it’s about building a comprehensive framework around it:
Data Quality: Make sure you have high-quality, domain-specific data to train the model. Fine-tuning requires an adequate amount of relevant, clean data to be effective.
Supporting Tools: Leverage platforms like Google Cloud's Vertex AI or Meta’s LLaMA fine-tuning studio to streamline the process and ensure you're equipped to manage, monitor, and evaluate your fine-tuned models effectively.
Continuous Improvement: Fine-tuning isn’t a one-time process. It’s iterative. As your model processes more data and interacts with live users, regularly updating and refining the model is key to maintaining accuracy and efficiency.
Conclusion
Fine-tuning remains an essential tool in specific cases, particularly when personalization, user-specific optimization, or risk mitigation are priorities. However, the advancements in AI models have made it less critical for more generalized tasks. CEOs should evaluate their use case, data availability, and desired outcomes when deciding whether to invest time and resources into fine-tuning AI models.
Call to Action
If you want to leverage fine-tuning for your business or need expert guidance on crafting a tailored AI strategy, Pipemind is here to help. We specialize in developing custom solutions, from model fine-tuning to full-scale AI deployments that ensure your technology works seamlessly with real-world data and use cases. Reach out to Pipemind today to explore how we can enhance your AI capabilities and drive better outcomes for your business.
Comments